Time-resolved X-ray tomographic microscopy provides new opportunities in the volumetric investigation of dynamic processes. Full exploitation of these new capabilities is currently still hindered by the lack of efficient post-processing approaches capable of handling TBs of noisy datasets. A deep learning based reconstruction and classification algorithm designed to reconstruct and segment dynamic processes within a static matrix with high efficiency is a solution to this issue. In a paper published recently in Scientific Reports, we demonstrate the advantages of the proposed approach on dynamic, time-resolved fuel cell data, for which the current data post-processing pipeline heavily relies on manual labor, typically limiting the experimental plans to just a small range of the full parameter space. The proposed approach reduces the post-processing time by a factor of 100 on a single GPU node readily available at most institutions. Furthermore, we demonstrate how the proposed training protocol for the classification network enables direct applicability of this new algorithm to other similar dynamic samples. This allows straightforward up-scaling to efficiently process larger data, opening up new possibilities in future dynamic X-ray tomographic investigations. The algorithm protocol flowchart is shown in the figure below.
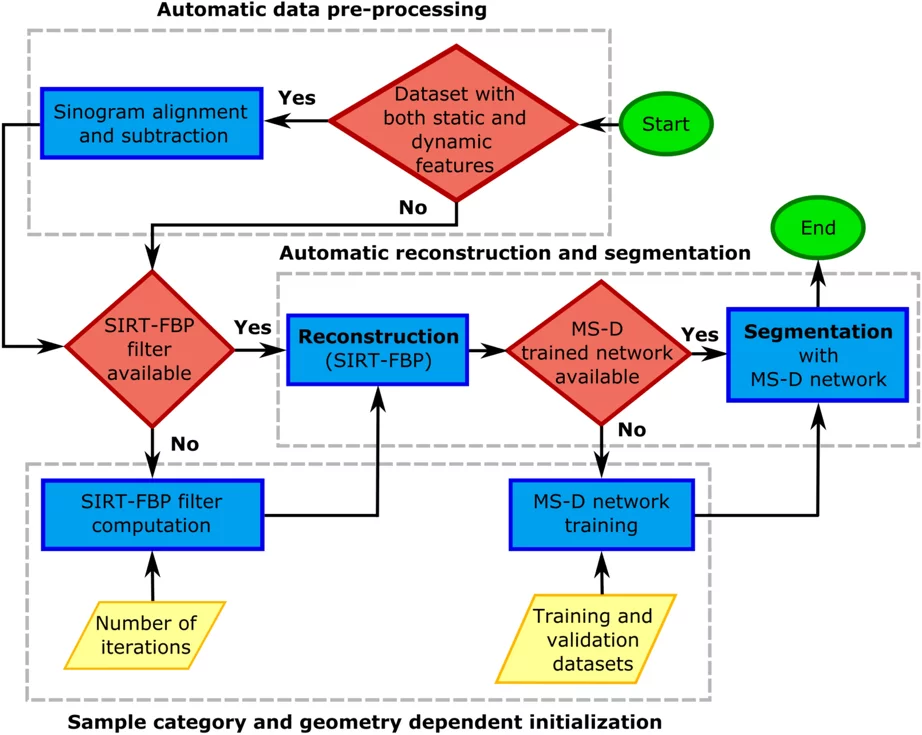
Original Publication
Deep learning based classification of dynamic processes in time-resolved X-ray tomographic microscopy
M. Bührer, H., Xu, A.A., Hendriksen, F.N., Büchi, J., Eller, M., Stampanoni, F., Marone
Scientific Reports 11: 24174 (2021)
DOI: 10.1038/s41598-021-03546-8